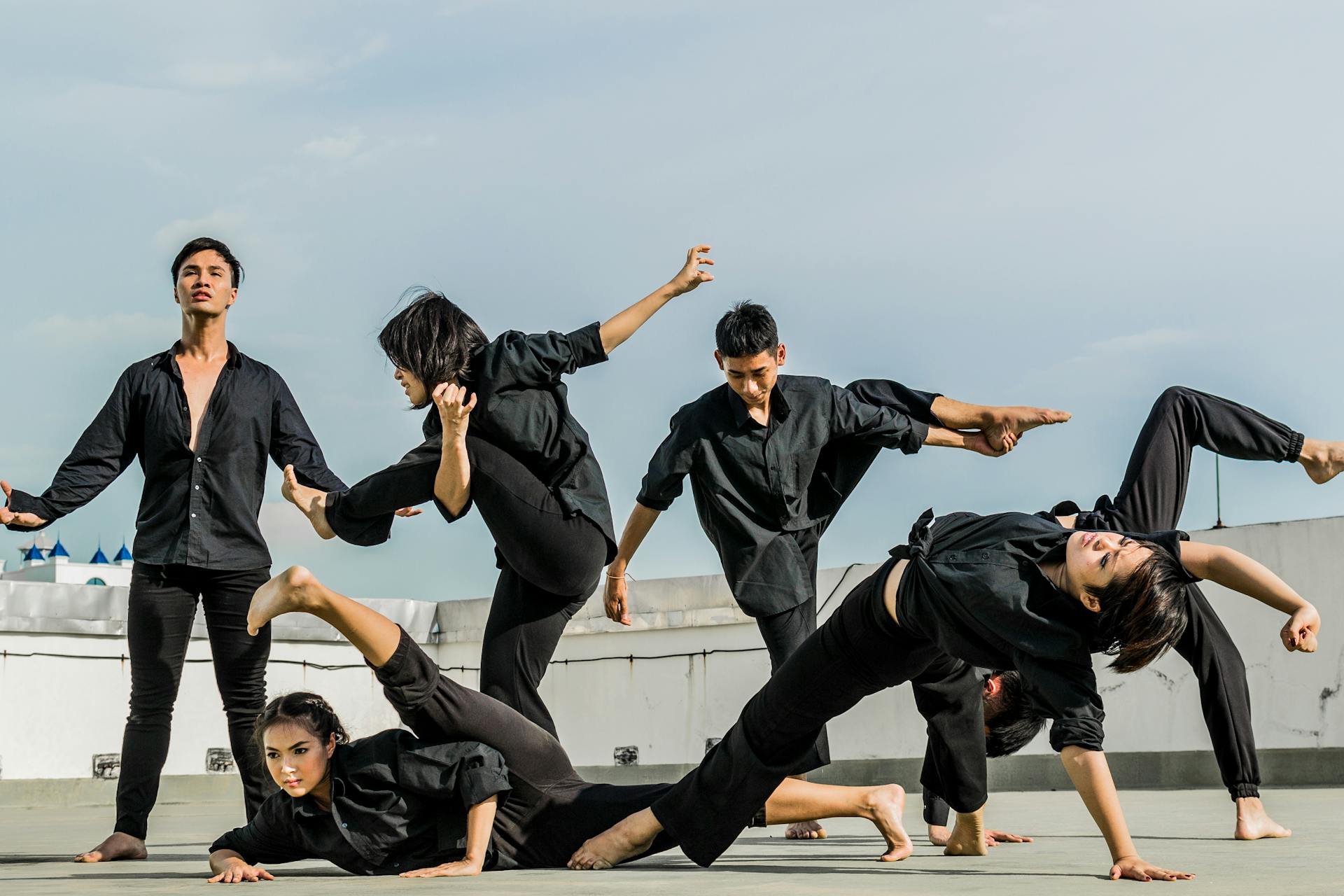
The Secret of 4x to 10x better Fraud Prevention Performance
Detecting potentially fraudulent payment requests and stopping them before they are accepted is an established trade. The standard tools of the trade are AI/ML scoring models and decision rules. This looks like an equal playing field for fraud prevention solutions. Yet it is not. One solution, IBM Safer Payments is standing out. By a whole lot.
The case
Here is an example in case: the major U.S. payment processor FIS replaced an incumbent scoring/rule solution with IBM Safer Payments. For Zelle mobile payments. Where fraud is abundant. Within 3 months of operations, they reduced fraud losses by 75% and false positives by 90%. That is 4x on fraud and 10x on false positive seven at the same time. On Nation scale. Real-time payments.
Secrets and the sauce
How is this possible? What’s the secret sauce? The silver bullet? The secret for this level of performance lies in the combination of functionality learned from hard-nosed experience in the industry. Safer Payments was designed by practitioners and AI geniuses.
Speed
Number one is speed. Fraud is fast and becoming faster, and fraud prevention must move at the speed of fraud. Lots of functions in Safer Payments allow for fast adaptation of the models and rules to emerging fraud patterns. First, all production data becomes immediately available for analytics and fraud countermeasure simulation. Minutes after a fraud trend emerges, integrated analytics isolate intelligence to construct fraud countermeasures. They use AI to propose countermeasures with completed simulation results on their impact. Customers use this to immunise their processing operations sometimes in even less than an hour. Not skipping a single step of a sound deployment process.
Another example in case: the national European payment switch QIWI processing 9 billion annual real-time payments found themselves overnight in an attack wave from a criminal group that was exploiting a weakness in their payment environment. Because hardware and software were involved, there was no quick fix of the vulnerability. It also affected all financial institutions in the region. SaferPayments assisted in finding a mechanism to differentiate the slight difference in fraudulent vs legitimate transaction execution. It took minutes to deploy and immunise their real-time payments. Most other financial institutions had to stop processing this transaction type at all for weeks.
Profiles
Number two is behavioural profiling. The key to high detection rates and low false positive rates is to differentiate criminal from legitimate payment behaviour. Often behaviour can only be detected by analysing large sequences of past transactions. And even more tricky, past behaviour must be compared to current behaviour. Current behaviour, and especially the current transaction to be assessed is only known now. Implying that past transactions must be assessed within the processing bracket of the current payment request. In numbers, for each payment transaction, for each behavioural feature to be extracted, up to hundred transactions must be assessed and compared to the current transaction in milliseconds. And that for multiple thousand payment requests per second.
Control
A third example in case: the French National payment switch STET used Safer Payments to save more than $1 billion in fraud losses at an ultra-low false positive rate of 1:1. The key to such low false positives is the flexibility in Safer Payments to not only look for incriminating evidence in behaviour, but also for exonerating evidence. When through changes in the legal system in France, legitimate payments at a large scale changed behaviour overnight, STET using Safer Payments was able to understand this to not be fraud and had avoided to intercept tens of thousands of legitimate transactions.